This post was published in 2022-07-09. Obviously, expired content is less useful to users if it has already pasted its expiration date.
Table of Contents
Title
Raphael, Christopher. "Automatic segmentation of acoustic musical signals using hidden Markov models." IEEE transactions on pattern analysis and machine intelligence 21.4 (1999): 360-370.
快速找到这篇论文:搜索「Automatic Segmentation of Acoustic Musical Signals Using Hidden Markov Models.pdf」
2022-03阅读论文并做的笔记
2022-03阅读论文并做的笔记(大概理解了70%,还剩一些细节没有搞懂)
2022-08-17补充内容
2022-08-17补充内容
上面的笔记是3月份写的,但我已经差不多忘记当时到底写了什么东西了,也暂时没有时间去纠正理解错误的内容。学习bayesian filtering之后,我对forward-backward有了更好的了解,现在临时写一个简短的笔记:
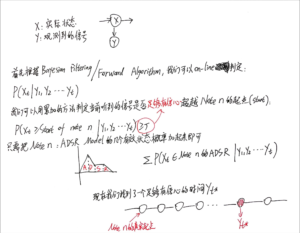
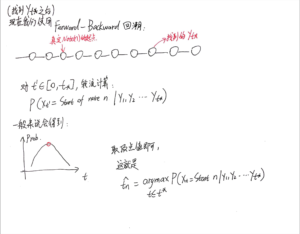
简单来说就是:
整个程序首先有一个bayesian filtering(Forward algorithm)从头到尾的跑,它是real-time的算法,讲究的是 实时 .
bayesian filtering计算实时的东西,但准确度有限(要捕获到 Note Start那一瞬间的30ms时间贞 还是有难度的,因为在那一瞬间bayesian filtering给出的置信值并不高)。我们往往会在note start之后过一段时间才通过bayesian filtering得到一个比较高的置信值,但此时早就过了note start,怎么把它从过去的时间里捞出来?使用forward-backward,把过去的时间剪下来单独进行计算,最后捞出note start对应的time frame. 由于forward-backward可以看作“非实时的离线计算”,计算它并不会影响我们bayesian filtering的运行。